Predictive analytics has become a cornerstone for making informed decisions and staying ahead of the competition. By leveraging artificial intelligence (AI) within Salesforce, businesses can harness the power of predictive analytics to forecast trends, optimize operations, and drive strategic initiatives. This article delves into the integration of AI for predictive analytics in Salesforce, offering practical applications and actionable steps for implementation.
The Power of Predictive Analytics
Predictive analytics uses historical data, statistical algorithms, and machine learning techniques to predict future outcomes. Key benefits include:
- Enhanced Decision-Making: Providing data-driven insights for strategic decisions.
- Improved Forecast Accuracy: Delivering precise sales, revenue, and demand forecasts.
- Optimized Resource Allocation: Ensuring resources are deployed where they’re needed most.
- Increased Customer Retention: Anticipating customer needs and behaviors to improve engagement and loyalty.
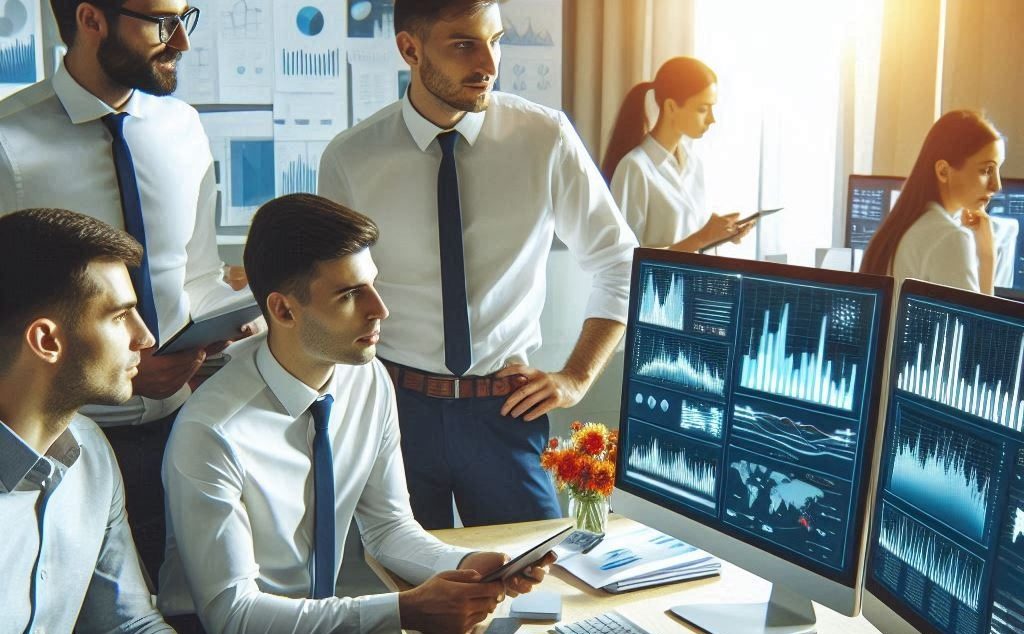
Integrating AI-Powered Predictive Analytics with Salesforce
Salesforce offers robust AI-powered tools through its Einstein platform, which can transform predictive analytics into actionable insights. Key features include:
1. Predictive Lead Scoring
Einstein AI can analyze historical data to score leads based on their likelihood to convert. This enables sales teams to prioritize high-value leads and focus their efforts more effectively.
2. Sales Forecasting
AI-driven sales forecasting provides accurate predictions by analyzing past sales data and market trends. This helps businesses plan more effectively and make informed decisions.
3. Customer Churn Prediction
Predictive analytics can identify patterns that indicate potential customer churn. By recognizing at-risk customers, businesses can implement retention strategies to prevent churn.
4. Demand Forecasting
AI can analyze market trends, seasonality, and historical sales data to forecast product demand. This ensures optimal inventory levels and efficient supply chain management.
5. Personalized Marketing
Predictive analytics can determine the best times and channels to engage with customers, allowing for highly targeted and personalized marketing campaigns.
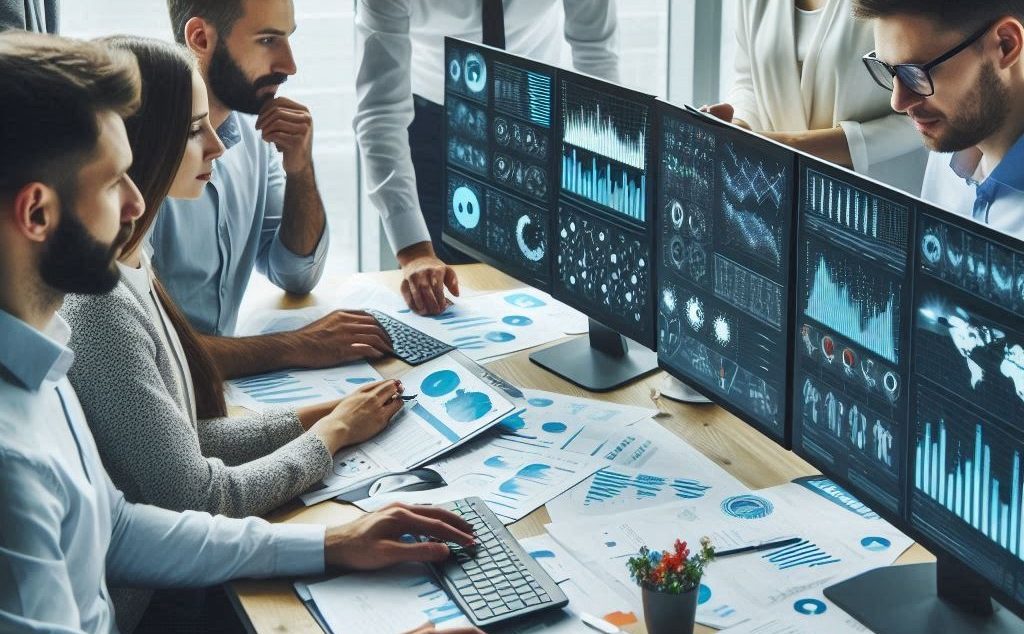
Practical Steps to Implement Predictive Analytics with AI in Salesforce
To effectively integrate AI-powered predictive analytics with Salesforce, follow these steps:
Step 1: Define Objectives and Key Metrics
Begin by defining the specific objectives you aim to achieve with predictive analytics, such as improving sales forecasting accuracy or reducing customer churn. Identify the key metrics that will help measure success.
Step 2: Enable Salesforce Einstein
Salesforce Einstein offers various AI-powered predictive analytics features. Enable Einstein Analytics and set up custom dashboards to track your key metrics and visualize insights.
Step 3: Integrate Data Sources
Ensure all relevant data sources, including CRM data, sales records, and market data, are integrated into your Salesforce environment. This provides a comprehensive dataset for accurate predictions.
Step 4: Train Your Team
Provide training to your team on how to use the predictive analytics tools in Salesforce. Ensure they understand how to interpret the data and apply the insights to their respective roles.
Step 5: Monitor and Refine
Regularly monitor the performance of your predictive analytics models. Use feedback and performance metrics to refine your models and strategies, ensuring continuous improvement.
Real-World Applications of Predictive Analytics in Salesforce
1. Boosted Sales Performance
A technology company used Einstein Predictive Lead Scoring to identify and prioritize high-potential leads. This resulted in a 15% increase in conversion rates and a 20% boost in sales revenue.
2. Improved Inventory Management
A retail chain implemented AI-driven demand forecasting to optimize their inventory levels. By accurately predicting demand, they reduced stockouts by 30% and minimized excess inventory.
3. Enhanced Customer Retention
A subscription-based service provider used predictive analytics to identify customers at risk of churning. By implementing targeted retention campaigns, they reduced their churn rate by 25%.
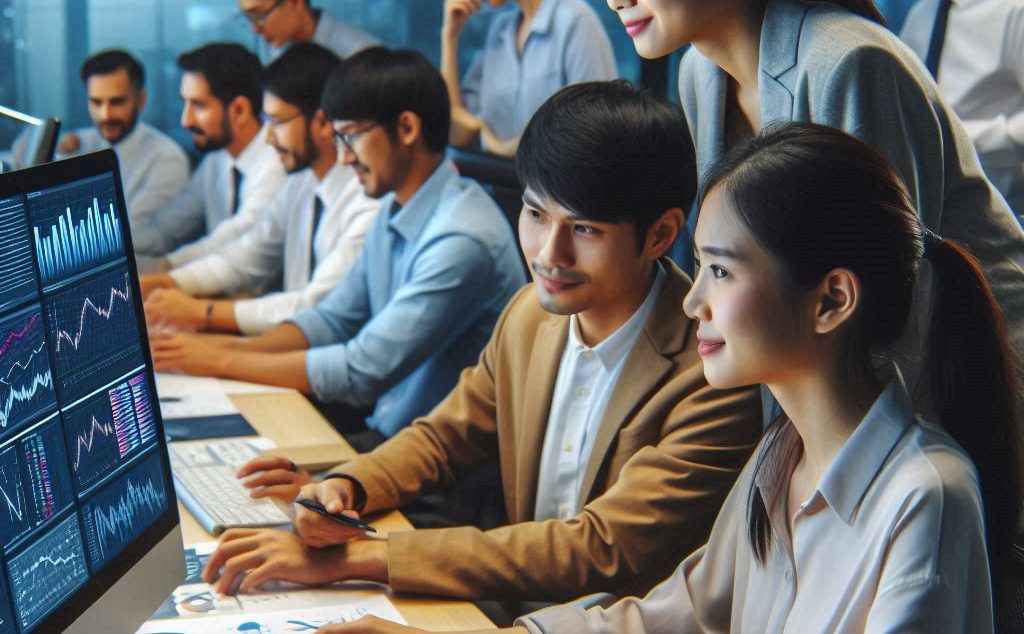
Best Practices for Implementing Predictive Analytics with AI in Salesforce
To maximize the benefits of integrating predictive analytics with Salesforce, consider these best practices:
- Focus on High-Impact Use Cases: Start with use cases that have the most significant impact on your business outcomes.
- Ensure Data Quality: Maintain clean, accurate, and comprehensive data to feed into your predictive models.
- Promote a Data-Driven Culture: Encourage a culture that values data-driven decision-making across your organization.
- Provide Ongoing Training: Regularly update training programs to keep your team proficient with the latest AI tools and techniques.
- Continuously Improve Models: Monitor the performance of your predictive models and refine them based on new data and feedback.
Conclusion
Leveraging AI for predictive analytics in Salesforce empowers businesses to make informed decisions, optimize operations, and achieve strategic goals. By integrating AI tools for lead scoring, sales forecasting, churn prediction, demand forecasting, and personalized marketing, organizations can enhance their performance and drive sustainable growth. Embracing these technologies and best practices will enable your team to stay competitive and proactive in an increasingly data-driven world.